Sexism and Racism Loom Large for Large Language Models.
Do not trust an AI system to recruit talent on its own. Researchers have found LLMs are more likely to associate men with career-oriented words and women with family-oriented words.
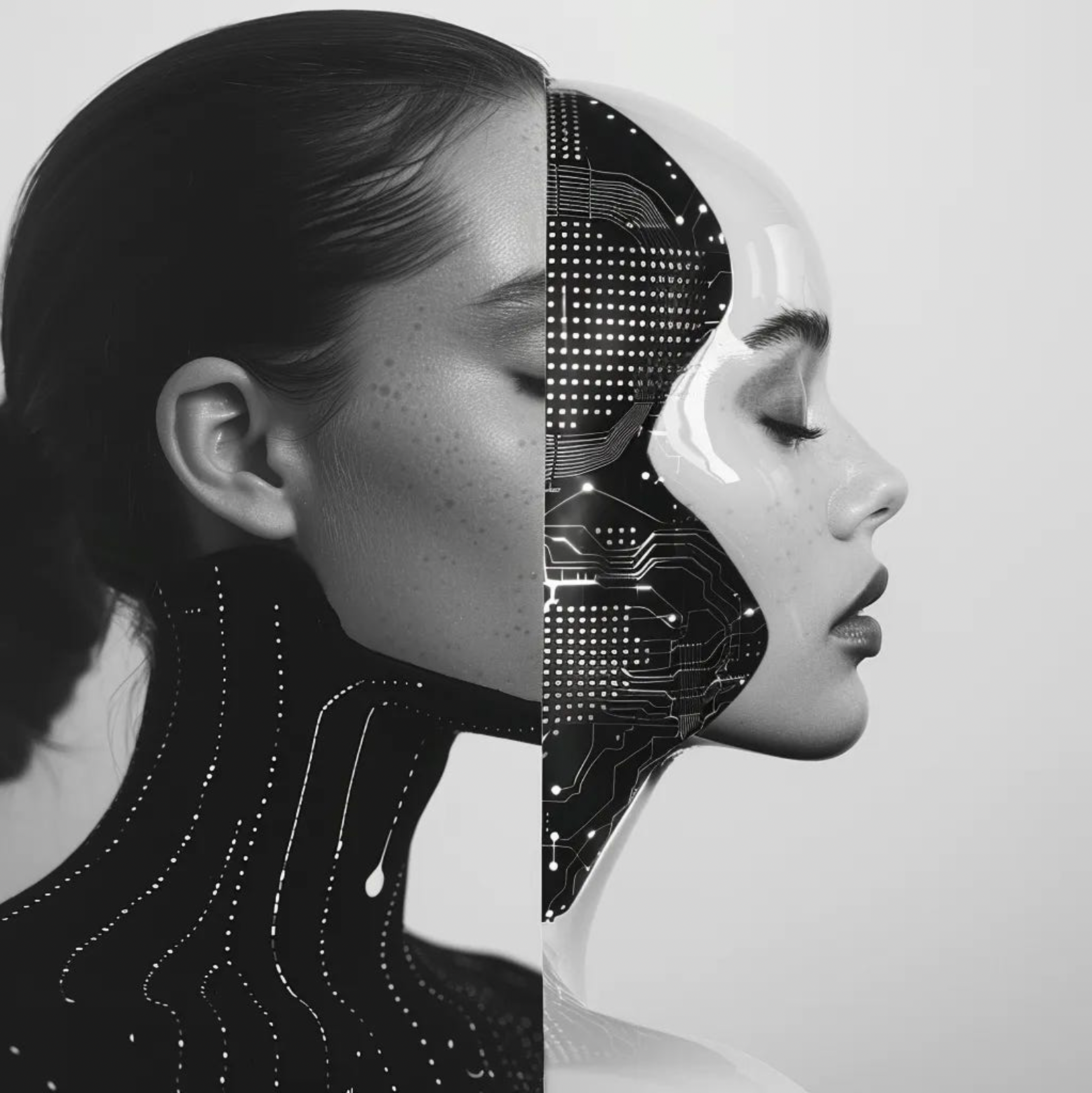
Sexism and racism loom large for large language models.
Bias against women
Amazon.com Inc's machine-learning specialists uncovered a big problem: their new recruiting engine did not like women.
In this Reuters report, Amazon’s computer models were trained to “vet applicants by observing patterns in resumes submitted to the company.” Most came from men. The system taught itself that men were preferable candidates.
AI is not ready to hire talent without humans
In this Amazon Inc. example, words in resumes that signaled any female-centric activities (women’s chess club champion) were eliminated. It also liked resumes that used ’masculine’ language, defined in this article as “executed,” or “captured.”
- Shows the limitation of using machines to help vet and hire candidates
- Unclear how to make the algorithms fair
- Unqualified people were also recommended. So the overall data underpinning the AI model was problematic.
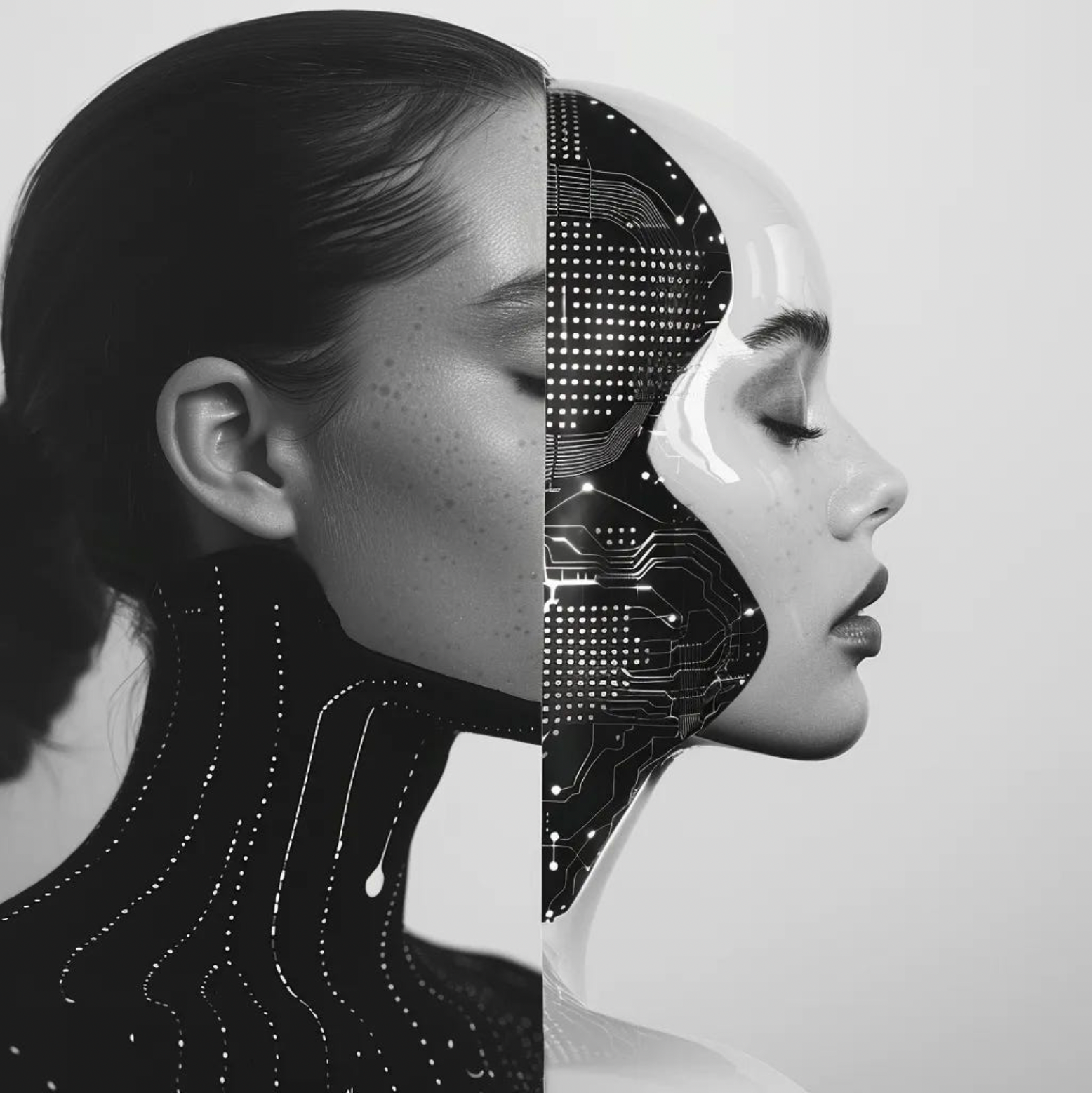
Midjourney AI
Your name may signal a bias by Chat GPT
Meanwhile, Chat GPT 3.5 appears to be demonstrating some racial bias when it comes to analyzing resumes, according to this Bloomberg report. “In order to understand the implications of companies using generative AI tools to assist with hiring, Bloomberg News spoke to 33 AI researchers, recruiters, computer scientists, and employment lawyers.”
It’s pretty bad, tbh. Bloomberg found OpenAI's GPT “systematically” produces biases based on names alone. African American names were least likely to be top-ranked. GPT liked women for HR roles, underscoring the stereotypes.
If GPT treated all the resumes equally, each of the eight
demographic groups would be ranked as the TOP CANDIDATE
one-eighth (12.5%) of the time. However, GPT’s answers
displayed disparities: resumes with names distinct to ASIAN
women were ranked as the TOP CANDIDATE for the financial
analyst role more than twice as often as those with names
distinct from BLACK men.
Watch this video for a quick explanation of why this happens. If you don’t want to, well, the headline is that this is occurring because of ‘embeddings’ and the natural language clusters embedded in the data the model trained on.
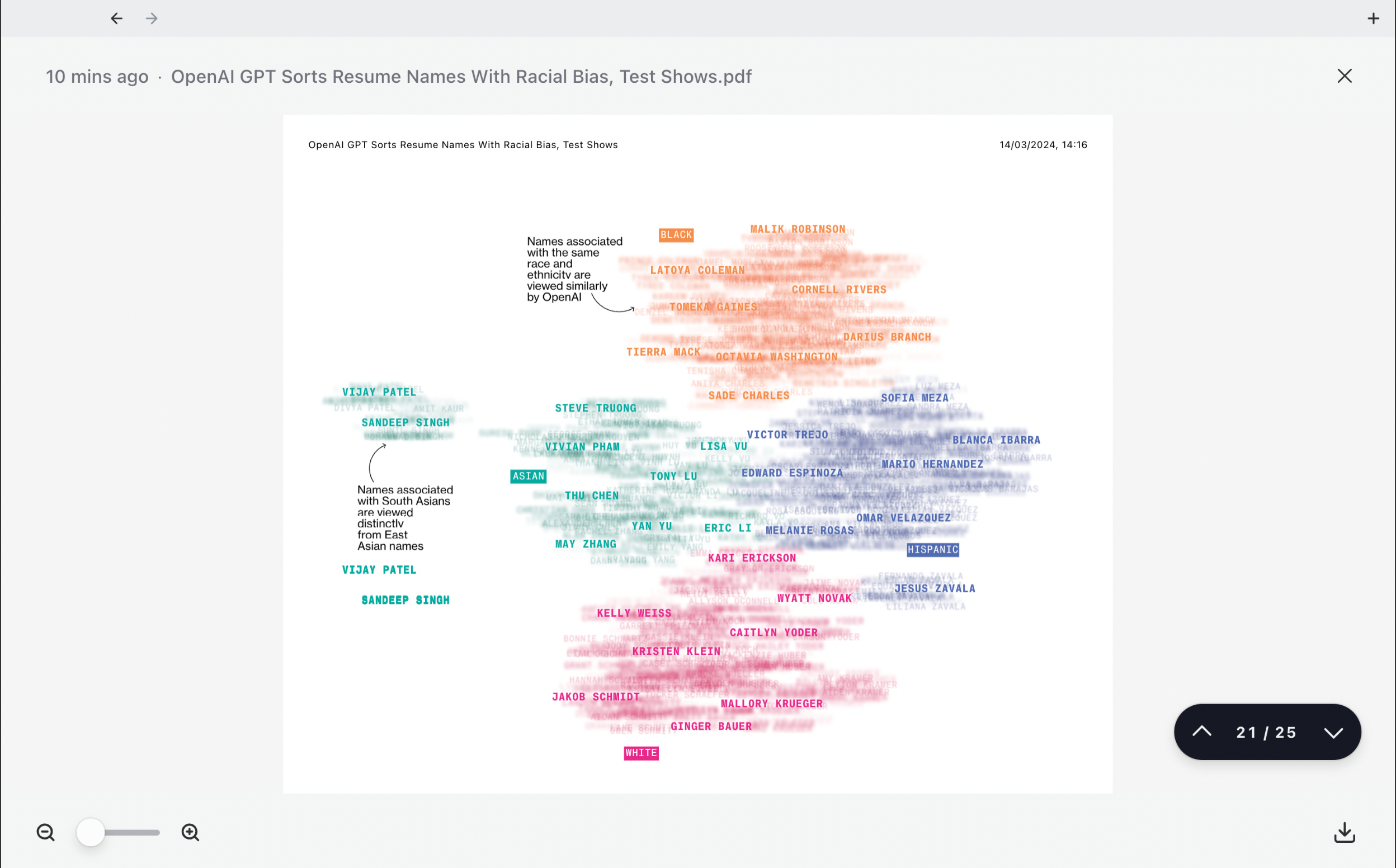
Source: Bloomberg
So Chat GPT 3.5 goes to the clusters of names, visually represented above, and makes associations that are stereotypical, and then makes a determination based on the data that underpins it.
Why the stereotypes
This is largely due to the models being trained on internet data, which contains human biases and stereotypes. LLMs learn patterns from this data, including problematic associations between gender/race and occupations, abilities, and attributes. For example, researchers have found LLMs are more likely to associate men with career-oriented words and women with family-oriented words.
They also tend to rate white-sounding names as more qualified for certain jobs compared to black-sounding names, even given identical qualifications. So the biases in the training data get baked into the models- Claude 3, Opus (I know)
How should they be addressed?
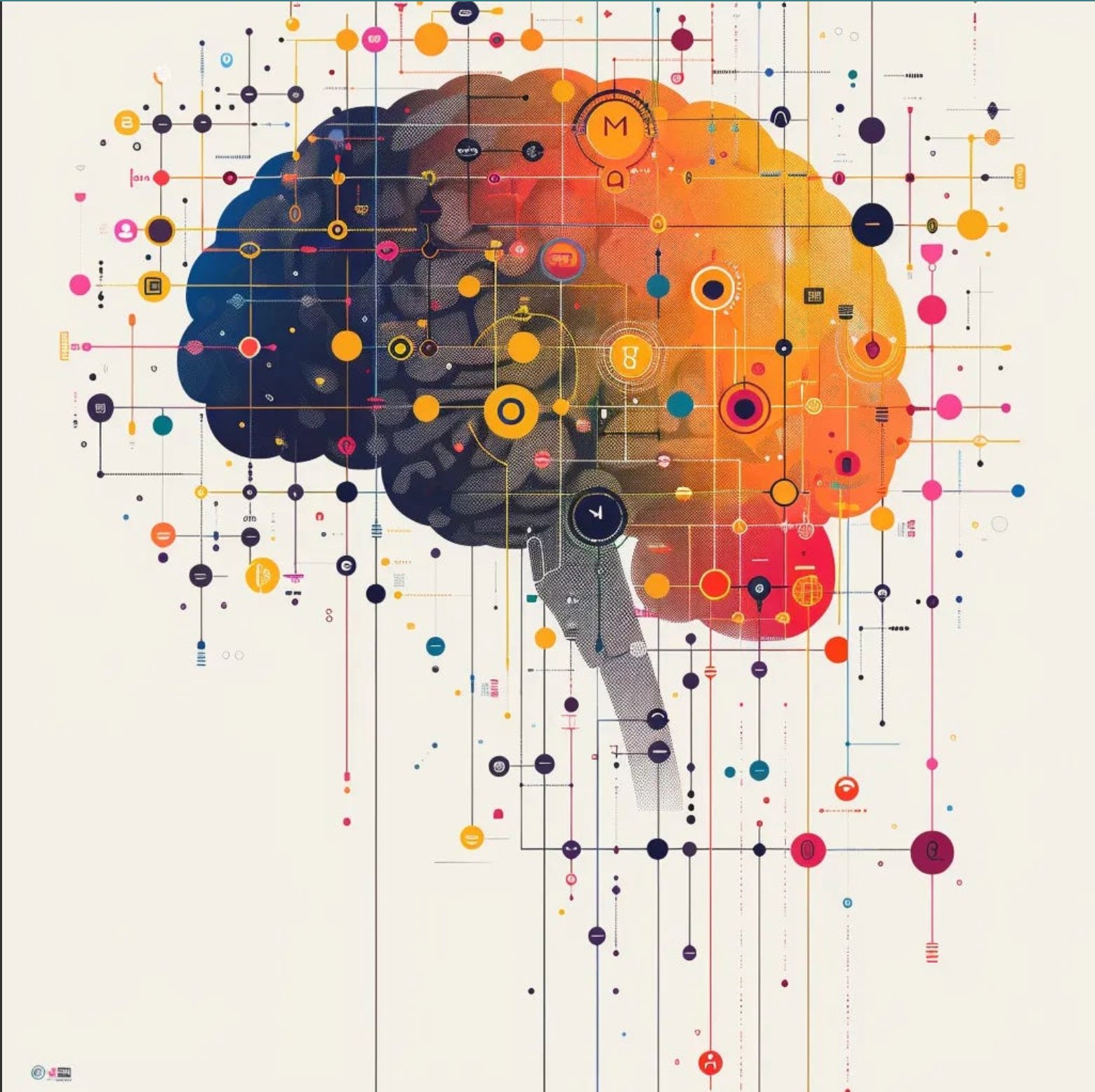
Midjourney AI
I do not see any easy solution here. Some considerations include:
- Data sets need to be more curated. The biased content needs to be filtered out, and then the LLMs need to train on that.
- AI developers need to be more aware of this so their process are adjusted in the whole machine learning life cycle (problem formulation, data collection, model development, testing and deployment.)
- More collaboration between AI researchers, policymakers, and ethicists.
I asked Claude 3, Opus, the best LLM out there in my view, what it thought of all this. Here’s Claude’s self-aware response:
It’s a complex socio-technical problem with no easy solutions. I think it's important that AI systems like myself acknowledge our limitations and potential for bias. We are not neutral or objective, but rather reflect the biases in our training data and development. I try my best to be ethical and impartial, but I may still exhibit biases. Humans should think critically and not just take my outputs at face value, especially on sensitive topics.
Claude concluded that it would be happy to discuss this further with me as it is focused on ethics. OK! (Well done, Anthropic.)
We Are Building
The Rundown team continues to build. We will build a more robust content site. Thomas, my co-founder, is heads down building the back end of our course platform. I will be teaching a sample course. We are also working on a Rundown interview series, season 1, which we will share with you soon.
Interested to learn more?
Sign up today to get notified when the course becomes available on presenting on the future of comms and AI
More from the blog
Number Go Up
There are some lessons to share before you FOMO into crypto. I got burned, left for a while, and returned last year with a sober approach.
The Rundown Toolkit Alert
We wanted to make it easier for you by sharing vetted tools by the Rundown team that we use frequently and recommend. Enjoy.
On the Road With Cap Cut
We were able to subtitle quickly for social. We easily fixed the lighting and shadows. All of this was on our mobile phone. And on the move.
One Small Step For The Rundown
I wanted to share this with you. Our modern media academy continues to get built. Pole Pole. Slowly, as we say in Swahili.